Your marketing strategy may be stuck in 2025.
While you’re carefully crafting segment-based campaigns, your competitors might be deploying AI agents that know exactly when your prospects are ready to buy — sometimes even before they do.
“Marketing based on segments is becoming outdated,” says Alex Lindahl, go-to-market (GTM) engineer at Clay. In this exclusive conversation, he challenges conventional marketing wisdom and introduces a revolutionary approach that’s generating 45% reply rates on cold outreach. He also reveals how AI orchestration is transforming customer engagement from a scheduled broadcast into an intelligent conversation.
As someone deeply involved in advancing these marketing frontiers, Alex is also set to share his insights and hands-on strategies during an upcoming workshop at the AI in Action roadshow in Atlanta in June 2025.
This isn’t just another AI think piece — it’s a practical roadmap for creating what Clay calls “Go-to-Market Alpha,” the competitive edge that comes from continual experimentation and adaptation.
This interview is part of G2’s Q&A series. For more content like this, subscribe to G2 Tea, a newsletter with SaaS-y news and entertainment.
To watch the full interview, check out the video below:
Inside the industry with Alex Lindahl
Many marketers are drowning in data but still struggling with actionable insights. In your experience working with Clay’s clients, how does an AI-first approach fundamentally change the way marketers should think about their customer data?
When thinking about data utilization, it’s important to consider the different types of data that are out there. We have structured data, found in databases, CRMs, and product usage metrics. Then there’s unstructured data scattered across the web in the form of blog posts, job postings, financial reports, and countless other sources. So you need to recognize that each company has a unique ideal customer profile (ICP) and distinct personas that require specific data sets. So the key is figuring out where you can source that data, both internally and externally.
With the advent of AI, we can now deploy agents to gather unstructured data from around the web, analyze it effectively, extract meaningful insights, and develop actionable recommendations. The real power comes from bringing these two elements together and combining diverse data sources with AI capabilities to accelerate understanding and decision making.
Historically, data utilization has largely been manual, often limited to basic segmentation for strategic decisions. But today, we can apply AI across different parts of the GTM funnel and business processes to dramatically improve how we gather information, analyze patterns, and take action based on comprehensive data insights.
Given that the gap between generating insights and actually implementing them is a constant challenge in marketing, what frameworks do you recommend for organizations to turn AI-generated insights into concrete business actions systematically? Are there specific tools or processes that you’ve seen work particularly well?
Yeah totally. We think of Clay as a system of action. So within Clay, you can have different columns powered by AI or data sources, and AI can analyze and structure this data effectively. For instance, if you’re targeting a VP of Marketing, AI can align the right case study or blog post specifically for that person. The key is applying AI in a structured way across different workflow types.
AI agents also come into play here. Historically, ICP personas, and marketing and sales plays have existed separately without a cohesive timing element connecting them. What I’m now calling “ideal customer signals” defines specific indicators that reveal potential business opportunities or challenges where your product can provide value. This approach moves us away from traditional segments toward signal-based engagement.
And so I came up with this framework called “DRIVE” that structures this approach.
D – Detect: Identify the signal and determine how to detect it and how frequently to search for it.
R – Review: Analyze what the signal implies. You can leverage AI here — for example, if a company is hiring DevOps engineers, AI can help conclude that they’re scaling their engineering team. This review helps determine whether the signal warrants action.
I – Identify: Uncover challenges the company may face in reaching its goals. AI excels here when trained on your own data, blog posts, case studies, and their outcomes.
V – Value: Align your solution’s value to the specific situation the company is experiencing.
E – Execute: Take appropriate action based on the signal.
The ideal implementation involves always-on signals constantly monitoring defined account lists. This allows you to target specific accounts precisely when relevant signals emerge, indicating timely opportunities for engagement.
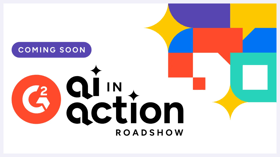
Transform your AI marketing strategy.
Join industry leaders at G2’s free AI in Action Roadshow for actionable insights and proven strategies to reimagine your funnel. Register now
Many marketers are experiencing “prompt fatigue” from continually refining their AI instructions. What frameworks or approaches do you recommend for creating more sustainable, reusable prompt strategies?
I personally find prompt engineering incredibly fun and powerful. Especially as a non-technical person, because now basically everyone’s becoming an engineer. You can use platforms like Lovable to even prompt your way to creating an application. But in the GTM world, there are tons of prompts that you can create, tons of prompts that you can action.
So, how do you go from just taking a prompt and refining it over time?
There are several approaches to create repeatability with your prompts. One method is developing a custom GPT by embedding your refined prompt into a specialized model, enriching it with contextual materials and learning resources. This creates a reusable tool that consistently generates marketing assets or provides competitive analysis without requiring you to recreate the prompt each time.
Another implementation strategy involves AI agents. At Clay, we utilize an AI agent that executes custom prompts for research purposes. For instance, you might direct the agent to determine whether a specific company has a shopping cart on its website. The agent will visit the site, analyze it, and return with a definitive answer or relevant explanation.
The real power comes from applying this capability across multiple accounts — whether hundreds, thousands, or even hundreds of thousands — allowing you to scale your prompt’s functionality efficiently.
The third approach integrates these prompts directly into operational workflows. Consider a simple example: When an inbound lead arrives, you could apply a prompt to categorize the person’s title based on seniority or job function. A subsequent layer might then recommend the most appropriate case study to send them. This creates a sequence where AI interventions lead to progressively better data or action outputs.
The process requires some initial investment in creating, refining, and testing your prompts. However, once optimized, you can deploy them through custom GPTs, AI agents, or workflow integrations where they’ll function continuously within their designated context. This eliminates the tedious cycle of repeatedly copying and pasting prompts into ChatGPT, which quickly becomes exhausting.
Your “Build the Prompts & Action the Insights” session in the “AI in Action Roadshow” promises to help marketers rethink their current approach. What transformation do you typically see in marketers’ thinking from the beginning to the end of this workshop?
I think what a lot of people are realizing is that marketing based on segments is becoming outdated. The new approach is using signals to align the right marketing or sales play to your ICP or persona. So, we are seeing a shift in thinking — signals are now taking precedence over segments.
Rather than creating conventional campaigns where you might send one message this week and a different one two weeks later with perhaps a few variations, we’re now focusing more on listening to the market, accounts, or individuals. This allows us to apply actions or deliver marketing messages precisely when they’re most likely to resonate with the recipient. That’s one of the key insights people typically take away from our workshop.
Another realization is that prompt engineering, while sounding complex or intimidating, is actually quite accessible. You only need to understand some fundamentals to improve your prompts over time. You can begin with a basic prompt and simply ask AI how to enhance it or what elements might be missing. This back-and-forth dialogue with the AI helps refine your prompt based on its recommendations, and you can incorporate these suggestions or even have the AI rewrite the prompt entirely for you.
You just need to ask the right questions and think about what the output is that you want, so you can articulate that clearly to AI. And perhaps the most significant takeaway is discovering how easily you can create a custom GPT, apply your refined prompt, and use it consistently to make that prompt repeatable.
This also accompanies the broader notion that everyone is essentially becoming an engineer today. You can create applications, build products, provide AI instructions, and develop AI agents — capabilities that weren’t previously accessible to non-technical people.
It’s exciting that individuals without technical backgrounds can now perform more technical functions by leveraging AI, making the entire process not just productive but enjoyable as well.
Alex Lindahl
GTM Engineer at Clay
Your workshop covers “AI orchestrations.” Could you explain how this concept specifically applies to marketing workflows and provide an example of how this has improved campaign performance?
So, a lot of the orchestrating of AI agents can be accomplished through several approaches. In Clay, it’s pretty straightforward — you set up a column that applies your AI agent instructions repeatedly across an account list. Alternatively, you could deploy an ambient agent that monitors for specific signals or implement a chat agent that engages with customers to qualify leads.
One of my favorite examples involves configuring a custom signal to track certain keywords on LinkedIn. When these keywords appear in posts, both the content and poster are automatically imported into Clay. The system then uses AI to analyze the person’s title, persona, and message content. Based on this analysis — particularly focusing on persona and title — it determines whether to proceed with message creation or take no action.
The next phase involves AI analyzing the post and completing a simple template like, “I just saw your post on [blank].” with AI filling in that blank space. This creates a personalized snippet that you can incorporate into your outreach. From there, an automation can trigger either a connection request, a LinkedIn message, or an email to that individual.
For this particular campaign, I actually saw a remarkable 45% reply rate, which is absolutely insane by industry standards. This success stems from incorporating timing elements and just-in-time messaging by using the detected signal to determine the optimal moment for outreach. Of course, we’re leveraging AI to filter prospects and ensure we’re connecting with the appropriate seniority levels.
As you can see, this approach significantly boosts the overall campaign effectiveness because it’s more personalized, timely, and fundamentally more relevant to what that person is thinking about or experiencing in that specific moment.
For people who recognize the potential of an AI-first approach but work in traditional organizations, what practical advice would you give to someone who wants to champion these methods within their team but faces institutional inertia or skepticism from leadership?
I would say first develop some level of comfort and knowledge around AI itself. Take a task you believe could be automated and experiment with tools like ChatGPT or a custom GPT to streamline it. This initial step focuses on improving your own productivity while gaining valuable prompting experience and understanding what’s possible and what isn’t.
Through this process, you’ll naturally become more efficient and develop shortcuts for specific tasks, such as converting call transcripts into follow-up emails. This hands-on experience builds your foundation in prompt engineering.
Next, identify a project within your team where you can create a prompt that’s going to help accelerate that project and move it forward. And through that, you’re going to start to expose your teammates to prompting and AI, and they’ll probably be pretty surprised. Once you’ve established this foothold, determine how to integrate AI into your team’s regular operations — their daily or weekly workflows. This gradual expansion demonstrates value at increasingly broader levels: first personal productivity, then project acceleration, and finally, team-wide implementation.
These progressive successes provide the evidence needed to build a compelling business case. You can now make informed recommendations based on demonstrated outcomes: “We’ve successfully applied this at individual, project, and team levels. Let’s scale this approach to other departments to achieve similar return-on-investment (ROI), productivity gains, and efficiencies.”
If readers could take away just one insight from this article, what would you want them to remember?
I’d say the most important takeaways are really two things. First, have fun with AI because AI exploration can be genuinely enjoyable. Second, experiment with your own use cases and within your company’s GTM strategy.
At Clay, we’ve developed this concept of “Go-to-Market Alpha,” which is essentially finding ways to do things differently to gain competitive advantages. You can’t discover these advantages without experimenting. Some experiments won’t show ROI, but through this process, you’ll discover approaches that work remarkably well.
The old playbooks aren’t working because we’ve stopped experimenting. We need to continually experiment to find that new playbook, that new wedge, that new ‘Go-to-Market Alpha’.
Alex Lindahl
GTM Engineer at Clay
So keep experimenting both personally and with your team and company. You’ll start to discover different pockets of “alpha” — competitive advantages that benefit both you and your organization.
If you enjoyed this insightful conversation, subscribe to G2 Tea for the latest tech and marketing thought leadership.
Follow Alex Lindahl on LinkedIn to learn more about signal based marketing.
Edited by Supanna Das